net.load_state_dict(torch.load(model_weight_path, map_location='cpu'))
时间: 2024-05-29 21:12:44
浏览: 163
This line of code loads the state dictionary of a pre-trained [pytorch](https://geek.csdn.net/educolumn/1309512bb95bbd063a82fd0157e558d8?spm=1055.2569.3001.10083) model from a specified file path, with the option to specify the device loc[ati](https://geek.csdn.net/educolumn/150d7073277950db5f09620704e791cf?spm=1055.2569.3001.10083)on for the loaded model.
- `torch.load(model_weight_path)` loads the state dictionary from the file path specified by `model_weight_path`.
- `map_loc[ati](https://geek.csdn.net/educolumn/150d7073277950db5f09620704e791cf?spm=1055.2569.3001.10083)on='cpu'` specifies that the loaded model should be [move](https://geek.csdn.net/educolumn/1017fe3bbe75a8f0c3f4fe48f3af73ee?spm=1055.2569.3001.10083)d to the CPU if it was originally trained on a GPU. This is useful if you do not have access to a GPU or if you want to test the model on a CPU.
Assuming `net` is the [pytorch](https://geek.csdn.net/educolumn/1309512bb95bbd063a82fd0157e558d8?spm=1055.2569.3001.10083) model you want to load the weights into, this line of code would load the pre-trained weights into `net`.
相关问题
net.load_state_dict(torch.load(model_weight_path, map_location='cpu'))什么意思
这行代码的意思是从指定路径(`model_weight_path`)加载模型的参数(state_dict),并将它们存储在
net.load_state_dict(torch.load(model_weight_path, map_location='cpu')) # for param in net.parameters(): # param.requires_grad = False # change fc layer structure in_channel = net.fc.in_features net.fc = nn.Linear(in_channel, 5) net.to(device)
在段代码中,`net` 变量应该是一个 PyTorch 模型实例,而 `model_weight_path` 是指模型的权重文件路径。错误信息 `NameError: name 'net' is not defined` 可能是由于在此之前没有定义 `net` 变量,或者定义了但是没有正确初始化。你需要通过以下方法之一来解决这个问题:
1. 确保在使用 `net` 变量之前已经定义它,并且正确地初始化了它。例如:
net = MyModel() # 使用自己定义的模型类初始化 `net`
net.load_state_dict(torch.load(model_weight_path, map_location='cpu'))
in_channel = net.fc.in_features
net.fc = nn.Linear(in_channel, 5)
net.to(device)
```
相关推荐
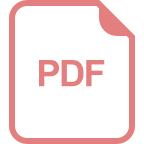
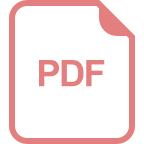
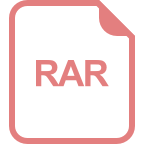











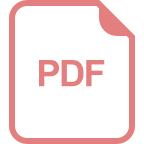
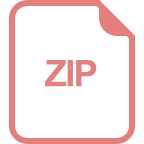